Unlike bank robbers, hackers do not attack with their weapons drawn. They use holes in networks and missing patches, or camouflage their malware in such a way that either employees end up opening an infected attachment, or purely signature-based antivirus solutions do not detect it. After all, cybercrime is a billion-dollar business. The developers of encryption Trojan Gandcrab, for example, have collected ransoms amounting to more than two billion US dollars. In order to protect their revenue streams, malware developers work day in and day out to ensure that antivirus solutions do not detect the malicious code. To do this, they rely on Darknet services that regularly run fully automated checks on whether security solutions are detecting their malware. In the event of detection, the malicious code is automatically modified with “packers” or “crypters” until it is no longer detected. Hence hackers are able to create new malware samples at almost a minute’s notice. To keep up with this increase in pace, more and more providers like us at G DATA are using AI technologies to detect these packed malware samples quickly and effectively. We have explained how DeepRay works and spoils things for cyber criminals in numerous previous blog posts.
Taking the next step
However, it is no longer sufficient to detect only known and repeatedly repackaged malware. Attacks are becoming more and more targeted, and are increasingly directed against companies. Instead of sending out mass emails, cyber criminals are targeting their victims. For example, they use social media platforms to search for events that an employee of a company under attack has attended. Then they refer to these events in a targeted “spear phishing” email. This is extremely difficult for the victims to see through because it looks very authentic. In some cases, the process of generating targeted emails is already being automated. For example, reference is made to email communication that the attackers have captured from an already infected system belonging to another organisation. Such attacks are called “dynamite phishing”.
In their attacks, criminals are increasingly using previously unknown malware, or specialised malware of which there are only a few known samples. Alternatively, they combine legitimate tools for a chain of attacks that has a damaging effect overall (“living off the land”).
The graph below shows such an example. A user has been persuaded by an attacker to open a malicious download from a web browser. Or he has been the victim of a vulnerability exploited by a so-called exploit. The harmful download “malware.exe” is clearly ransomware that encrypts the user's data and demands a ransom for decryption.
This is shown by the fact that the process first opens an instance of the system tool “bcdedit” in order to deactivate the Windows recovery function. At the same time, another system tool called “vssadmin” prevents the creation of so-called “volume shadow copies” that can be used to recover files that have recently been accidentally overwritten. The malware then begins to encrypt files in the user directory. All in all, this is a path typical of ransomware. However, the individual steps in this attack do not look suspicious. There can sometimes be several days or weeks between the individual phases.
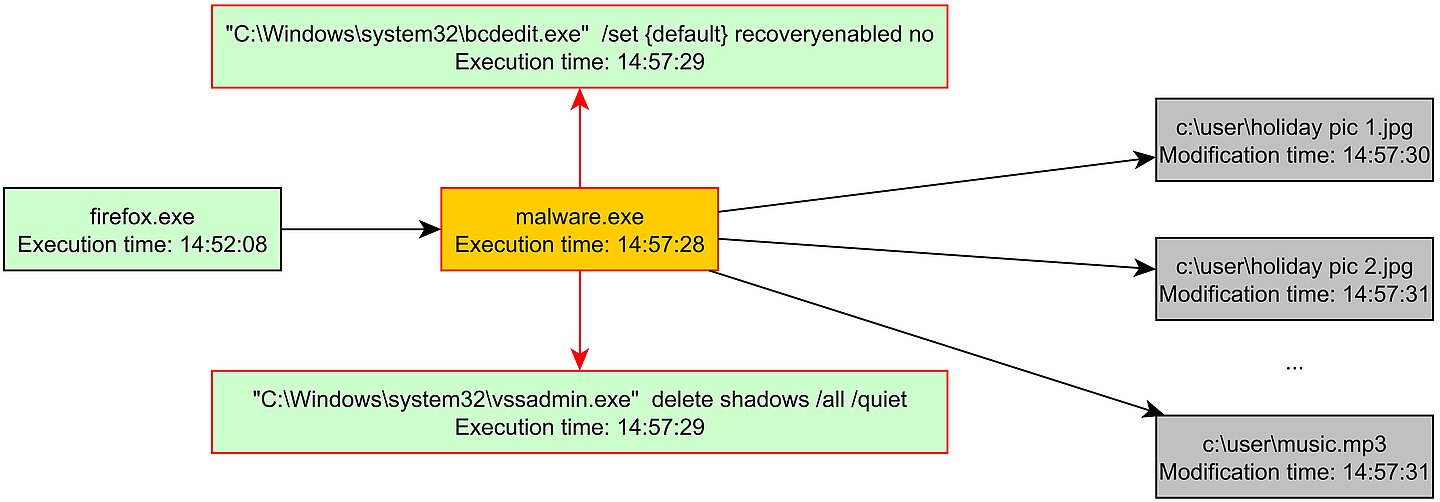
Behaviour monitoring can be used to identify such malicious process chains. This analyses the behaviour of processes on the computer and monitors, for example, changes in the file system and registry - especially those involving suspicious locations such as the auto start folder. However, existing behaviour-based detection approaches attempt to translate potentially threatening behaviour into numerical values. They thus define a degree of “badness”. However, when the numerical value is aggregated, information is necessarily always lost, resulting in a degree of uncertainty. Although this makes it possible to detect even unknown malware families, the technology is more prone to miscalculations than other detection methods. Either the detection threshold is set so high that hardly any malware is detected, or the threshold value is set so low that false alarms - called false positives - frequently occur.
False positives occur particularly frequently in the corporate environment due to the use of specialised software. At the same time, they are a particular problem there. A familiar phenomenon is that people who receive pointless warnings begin to ignore them. In the corporate environment this means that, if the elimination of false positives gives rise to higher costs than the presumed cost risk associated with an infection - at least in theory - then behaviour monitoring is often simply switched off.
It´s time for BEAST
To solve this problem, G DATA has developed BEAST. BEAST takes a radically different approach compared with previous behaviour analysis technologies. Instead of aggregating suspicious activities in a numerical value, the technology traces the processes in a graph. A performance-optimised graph database that runs locally on the computers of G DATA customers was developed for this purpose. The advantage of the graph database is that it captures a complete picture that can clearly identify threats. BEAST can also detect much more complex, lesser known combinations of processes. Information on current threats is collected and the relationships between different samples and IoCs are analysed in a large graph database, which grows by several million nodes and edges every day. The result is a comprehensive picture of the current threat situation. Even malware that distributes each individual activity to a separate process can be identified. In the second part of the blog series, we explain how BEAST works and what insights the experts gained during the development of BEAST.